Difference between revisions of "Environmental-driven Massing Based on Machine learning"
Abel Maciel (talk | contribs) (→Conference Paper) |
|||
Line 5: | Line 5: | ||
[[Category:Conferences]] | [[Category:Conferences]] | ||
[[Category:Book]] | [[Category:Book]] | ||
− | [[DC I/O 2022]] Paper by [[Hangchuan Wei]], [[Yota Adilenido]] and [[Richard Beckett3]]. https://doi.org/10.47330/DCIO.2022.EQAD1156 | + | [[DC I/O 2022]] Paper by [[Hangchuan Wei]], [[Yota Adilenido]] and [[Richard Beckett3]]. https://doi.org/10.47330/DCIO.2022.EQAD1156 | [[File:YouTube.png |Left|30px|link=https://youtu.be/n_XrzWdqQl4]] | [[File:PDF-Icon.png |Left|25px|link=https://www.dropbox.com/sh/8y9bge690wl2t2k/AABASKrdPFu3v_UOH5e9xfjra?dl=0&preview=DCIO2022_S1-2_EQAD1156.pdf]] |
Line 19: | Line 19: | ||
This framework aims to provide an approach to achieve faster and better massing design. To reach this objective, there are three main steps: 1) firstly, a generative adversarial network (GAN) model is trained to get wind simulation results from the input site, 2) then, the possible boundaries of massing in different height are generated for exhaustive enumeration, 3) afterwards, run again the GAN wind simulation for the possible boundaries, 4) and finally an assessment method is put forward to obtain the ideal result for the site. | This framework aims to provide an approach to achieve faster and better massing design. To reach this objective, there are three main steps: 1) firstly, a generative adversarial network (GAN) model is trained to get wind simulation results from the input site, 2) then, the possible boundaries of massing in different height are generated for exhaustive enumeration, 3) afterwards, run again the GAN wind simulation for the possible boundaries, 4) and finally an assessment method is put forward to obtain the ideal result for the site. | ||
− | |||
− | |||
− | |||
− | |||
− | |||
− | |||
=Keywords= | =Keywords= |
Revision as of 10:31, 20 March 2023
DC I/O 2022 Paper by Hangchuan Wei, Yota Adilenido and Richard Beckett3. https://doi.org/10.47330/DCIO.2022.EQAD1156 |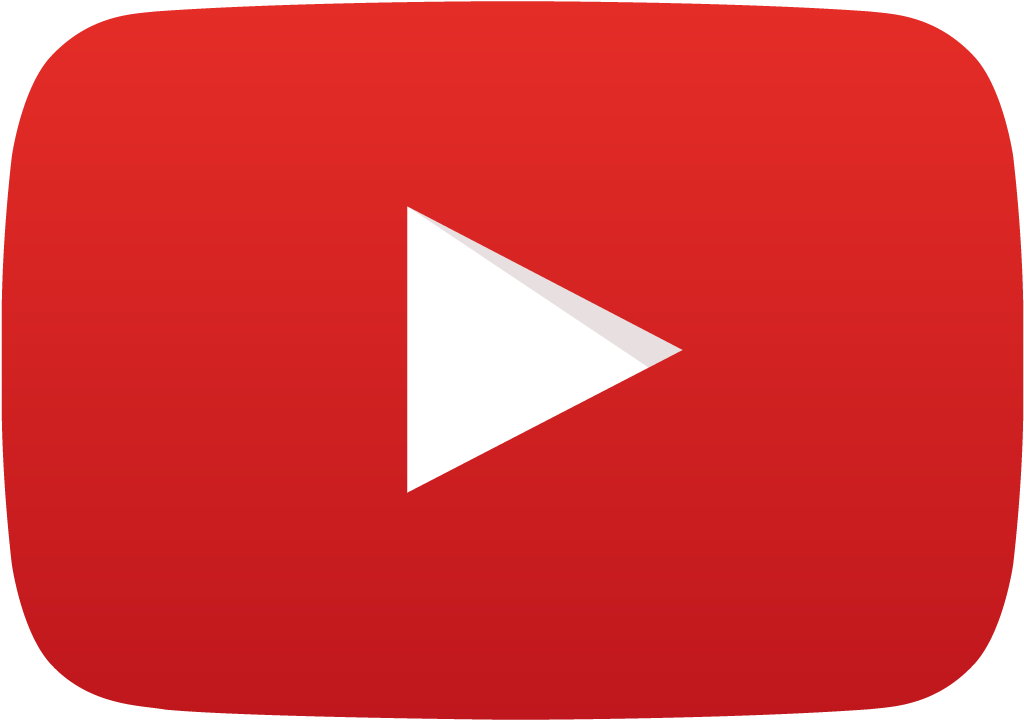
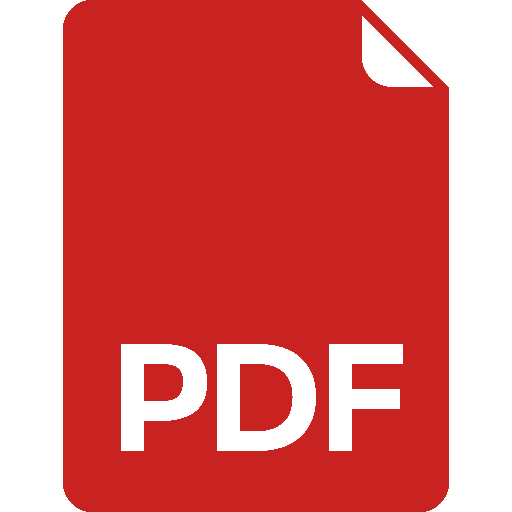
Contents
Abstract
In recent years, machine learning (ML) has received significant attention in the field of architectural design. This paper proposes a methodology for integrating ML with computational design to generate building massing based on environment, in this way, gives an outlook on the application of ML in architecture.
In the early stages of building design, a great deal of effort is often spent on specifying and designing building massing. In this process, the assessment of the building wind performance plays an important role. Compared to professional computational fluid dynamics (CFD) software, plug-ins based on rhino and grasshopper, such like Butterfly and Eddy3D, can well integrated into computational design process. But even then, these plug-ins are still limited because a lot of computing power and time are required to run the program.
This article provides an overview of a generative framework embedded with a ML approach to apply CFD in building design, finally results on a building massing with a balanced wind environment at the early stage of architectural design. This framework innovates the existing CFD simulation in following aspects: 1) ML-based simulation is timesaving, 2) this advantage allows the use of exhaustive enumeration to obtain the optimal solution, 3) this framework provides a good interface with computational design process with images as a medium, 4) therefore it is more flexible and operational.
This framework aims to provide an approach to achieve faster and better massing design. To reach this objective, there are three main steps: 1) firstly, a generative adversarial network (GAN) model is trained to get wind simulation results from the input site, 2) then, the possible boundaries of massing in different height are generated for exhaustive enumeration, 3) afterwards, run again the GAN wind simulation for the possible boundaries, 4) and finally an assessment method is put forward to obtain the ideal result for the site.
Keywords
Machine Learning, Generative Adversarial Networks, Generative Design, Computational Fluid Dynamics, Building Simulation.
Reference
DOI: https://doi.org/10.47330/DCIO.2022.EQAD1156
Bibliography
- BELÉM, C., SANTOS, L., AND LEITÃO, A. 2019. On the impact of machine learning. In International Conference on Computer- Aided Architectural Design Futures 2019 (CAAD Futures 19), 274-293.
- CHAILLOU, S. 2020. Artificial intelligence x architecture. In Architectural intelligence, Springer, 117-127.
- RUTTEN, D. AND MCNEEL, R. 2007. Grasshopper Robert McNeel & Associates, Seattle, WA.
- DEL CAMPO, M., MANNINGER, S., AND CARLSON, A. 2019. Imaginary Plans.
- GALANOS, T. AND CHRONIS, A., 2021. A deep-learning approach to real- time solar radiation prediction. In The Routledge Companion to Artificial Intelligence in Architecture, Routledge, 224-231.
- GOODFELLOW, I., POUGET-ABADIE, J., MIRZA, M., XU, B., WARDE- FARLEY, D., OZAIR, S., COURVILLE, A. AND BENGIO, Y., 2020. Generative adversarial networks. Communications of the ACM, 63(11), 139-144.
- ISOLA, P., ZHU, J.Y., ZHOU, T. AND EFROS, A.A., 2017. Image-to-image translation with conditional adversarial networks. In Proceedings of the IEEE conference on computer vision and pattern recognition, 1125-1134.
- KASTNER, P. AND DOGAN, T., 2022.A toolkit for decoupled door thermal comfort simulations in urban areas. Building and Environment, 212, 108639.
- LEYTON, M, 2003. A generative theory of shape (Vol. 2145). Springer, 366.
- MCNEEL, R., & OTHERS, 2010. Rhinoceros 3D, Version 6.0. Robert McNeel & Associates, Seattle, WA.
- SIDEFX SOFTWARES, 1996. Houdini, Version 19.0.455. Side Effects Software Inc, Toronto,
- SPACEMAKER, 2020. “Spacemaker”. https://www.spacemakerai.com/
- VAN DRUENEN, T., VAN HOOFF, T., MONTAZERI, H. AND BLOCKEN, B., 2019. CFD evaluation of building geometry modifications to reduce pedestrian-level wind speed. Building and Environment, 163, 106293.
- VLAD. 2019. Machine learning in Houdini because why not?. ODFORCE. December, 20, 2019, https://forums.odforce.net/topic/44527-machine-learning-in- houdini-because-why-not/
- ZHU, J.Y., PARK, T., ISOLA, P. AND EFROS, A.A., 2017. Unpaired image-to-image translation using cycle-consistent adversarial networks. In Proceedings of the IEEE international conference on computer VISION, 2223-2232.